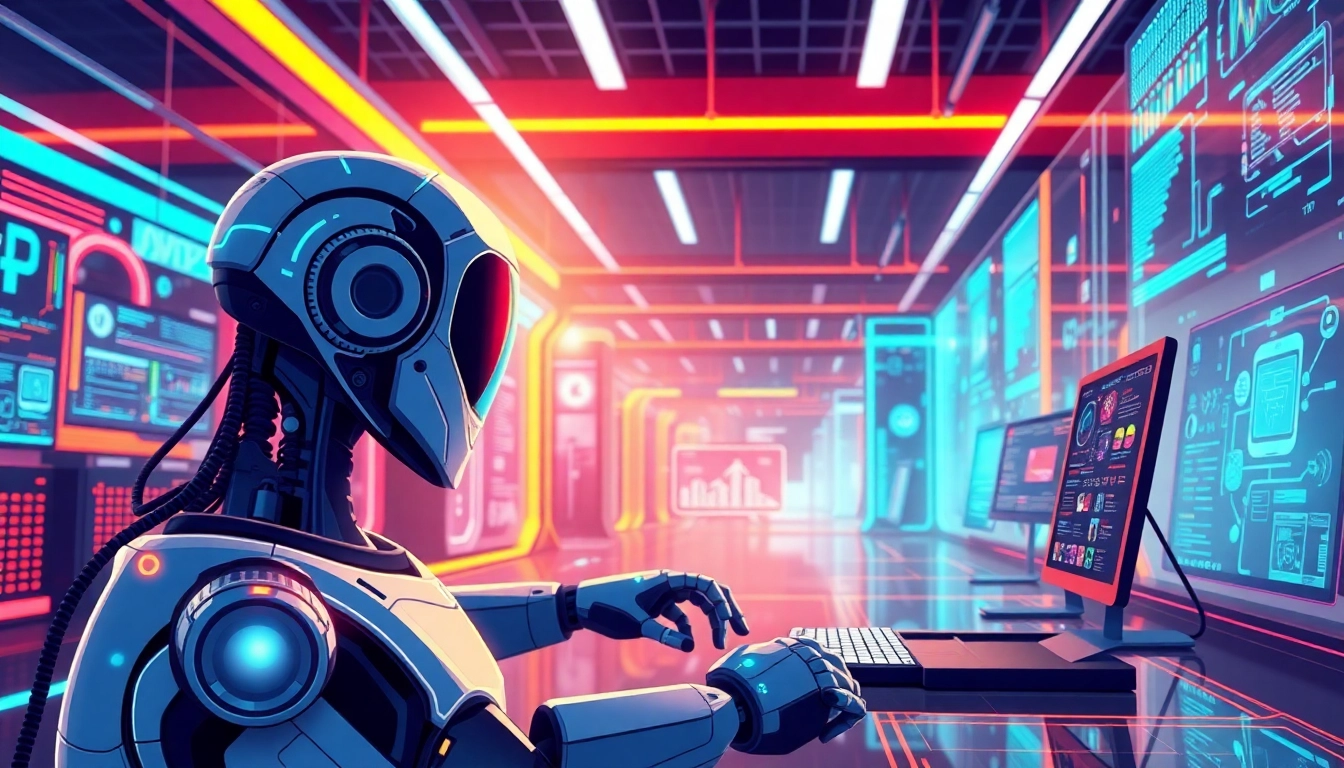
1. Introduction to Agentic AI
In the rapidly evolving landscape of artificial intelligence, one of the most cutting-edge concepts to emerge is Agentic AI. This innovative branch of artificial intelligence focuses on the capabilities of machines to operate autonomously, making decisions and taking actions to achieve specific goals without the need for constant human guidance. As industries grow increasingly reliant on intelligent systems, understanding the nature and potential of Agentic AI becomes crucial for businesses and technology enthusiasts alike.
What is Agentic AI?
Agentic AI refers to AI systems endowed with the ability to act independently, strategizing their course of action to achieve predetermined objectives. Unlike traditional AI paradigms, which often require explicit instructions for each task, Agentic AI leverages advanced algorithms, learning techniques, and data to navigate complex problem-solving scenarios autonomously. This capability allows the system to adapt to new situations, learn from experiences, and optimize decisions effectively.
The Evolution of Autonomous AI Systems
The journey towards Agentic AI began with the development of machine learning and traditional AI systems that could process data and provide insights based on analysis. As technology progressed, researchers and engineers sought to create systems that could not only analyze and infer but also act autonomously in real-world environments. The evolution of autonomous systems includes:
- Rule-Based Systems: Early AI systems operated on fixed rules and required human oversight to manage exceptions or novel situations.
- Machine Learning: Introduced algorithms that could learn from data, resulting in systems that improved over time but still needed human intervention for decision-making.
- Deep Learning: Utilizing neural networks for complex patterns, deep learning laid the foundation for more adaptive AI systems.
- Reinforcement Learning: By allowing systems to learn from their own actions and outcomes, this approach advanced the potential for autonomous decision-making.
- Agentic AI: The culmination of all previous innovations, where AI can set its own objectives, learn from a wider range of experiences, and make decisions without needing continuous human instruction.
Importance of Agentic AI in Modern Technology
Agentic AI is reshaping our world, influencing how businesses operate, individuals interact with technology, and entire industries function. Its importance can be highlighted in several areas:
- Efficiency: By automating decision-making processes, businesses can improve operational efficiency, reducing human error and accelerating workflows.
- Scalability: Enterprises can deploy agentic AI across various sectors, seamlessly handling numerous tasks without proportional increases in workforce size.
- Cost Savings: Reducing the need for human oversight can lead to significant cost savings in labor and error management.
- Innovation: Enhanced decision-making capabilities foster innovation by enabling organizations to test new strategies and ideas with decreased risk.
2. Key Features of Agentic AI
Autonomy in Decision Making
At its core, Agentic AI embodies the principle of autonomy. These systems can independently assess situations, determine optimal solutions, and execute actions to achieve set goals. This autonomy arises from multiple factors:
- Data Analysis: Agentic AI can quickly analyze vast amounts of real-time data, providing insights that drive decision-making.
- Predictive Modeling: By utilizing predictive analytics, Agentic AI anticipates outcomes based on historical patterns, informing its actions.
- Contextual Understanding: These systems are capable of understanding context, allowing them to make decisions that align with user or organizational goals.
Adaptability to Changing Environments
Environmental adaptability is a critical feature of Agentic AI. These systems can adjust their strategies based on real-time changes in data or conditions. This adaptability manifests in several ways:
- Real-Time Learning: They update their knowledge base continually, learning from new data and outcomes to enhance future decision-making.
- Scenario-Based Decision-Making: Agentic AI systems can simulate multiple scenarios, enabling them to choose the best course of action dynamically.
- Cross-Domain Functionality: These systems can apply learned behaviors from one domain to another, making them versatile across various applications.
Iterative Planning and Problem Solving
Problem-solving is central to the functionality of Agentic AI, utilizing iterative planning methods to approach complex challenges. This capability includes:
- Goal Setting: Agentic AI begins with a clear understanding of its objectives, translating them into actionable plans.
- Multiple Iterations: The systems evaluate their actions against benchmarks, iterating on their strategies until optimal results are achieved.
- Feedback Mechanisms: Continuous feedback allows these systems to refine their processes and improve their effectiveness over time.
3. Applications of Agentic AI
Real-world Use Cases of Agentic AI
Agentic AI is being leveraged across a variety of domains, showcasing its versatility and potential. Some key applications include:
- Healthcare: From diagnostics to personalizing treatment plans, Agentic AI systems can analyze patient data and suggest interventions.
- Financial Services: These systems can autonomously manage stock portfolios, automate trading strategies, and predict market trends.
- Supply Chain Management: AI can optimize inventory levels and streamline logistics by adapting to market demands.
- Smart Manufacturing: Agentic AI enhances production lines by predicting equipment failures and managing workflow adjustments.
Agentic AI in Business Operations
In the business landscape, Agentic AI is transforming operations by empowering businesses to automate crucial processes and enhance operational efficiency. Key areas of impact include:
- Customer Service: AI-driven chatbots and virtual assistants handle customer inquiries, providing solutions faster than human agents.
- Human Resources: AI systems streamline recruitment processes by analyzing candidates and matching them with role requirements.
- Marketing: By analyzing consumer trends, Agentic AI can create targeted marketing campaigns that adapt in real-time based on user interactions.
Impacts on Various Industries
Agentic AI’s influence extends over numerous industries, including:
- Retail: AI enhances personalized shopping experiences by recommending products that align with customer preferences.
- Transportation: Autonomous vehicles rely on Agentic AI for navigating complex routes and ensuring passenger safety.
- Education: Adaptive learning systems utilize AI to tailor educational content to individual student needs, thereby improving learning outcomes.
- Security: Surveillance systems enhanced by AI can predict and respond to risks in real-time, providing better security management.
4. Comparing Agentic AI with Generative AI
Distinct Roles and Capabilities
Understanding the distinction between Agentic AI and Generative AI is essential, as both serve unique functions within the AI landscape. Agentic AI focuses on:
- Action-Oriented Objectives: It aims to make autonomous decisions that yield specific actions.
- Real-Time Adaptability: Agentic AI dynamically adjusts its strategies based on environmental changes.
In contrast, Generative AI is predominantly concerned with content creation and involves:
- Creativity: It generates new text, images, or music based on patterns learned from existing datasets.
- Collaboration with Humans: Generative AI often requires human prompts to guide its outputs.
How They Complement Each Other
While Agentic AI and Generative AI have distinct roles, they can complement each other significantly. Businesses that deploy both systems can enhance their operational capabilities:
- Task Automation: Agentic AI can effectively handle operational tasks, leaving human employees free to engage with Generative AI for creative projects.
- Improved Customer Interactions: By integrating both types of AI, businesses can provide efficient service while generating personalized content for users.
- End-To-End Solutions: Combining the decision-making power of Agentic AI with the creativity of Generative AI empowers businesses to create comprehensive solutions that adapt to market needs.
Future Trends and Developments
As the field of AI continues to evolve, several trends surrounding Agentic AI and its relationship with Generative AI are emerging:
- Integration Across Platforms: Advances in technology will lead to more seamless integration between different AI systems, allowing businesses to derive value from both Agentic and Generative AI.
- Enhanced Learning Algorithms: As machine learning continues to mature, Agentic AI systems will become increasingly adept at contextual analysis and decision-making.
- Ethical Considerations: Ongoing discussions about AI ethics will shape how both types of AI are developed, governed, and implemented in society.
5. Challenges and Ethical Considerations of Agentic AI
Understanding the Risks of Autonomy
The development of Agentic AI brings significant benefits; however, it also poses potential risks that must be understood and managed. Concerns include:
- Decision-Making Errors: Autonomous systems may misinterpret data or situations, leading to poor decision-making consequences.
- Security Vulnerabilities: The dependence on AI systems can expose organizations to risks such as hacking or data breaches.
- Job Displacement: The automation of tasks traditionally performed by humans raises concerns about job loss and the future of work.
Ethical Frameworks for AI Decision Making
To navigate the ethical landscape of Agentic AI, businesses and developers must engage with existing ethical frameworks. These frameworks focus on:
- Transparency: Ensuring that AI decision-making processes are understandable and accountable.
- Fairness: Creating systems that do not discriminate or reinforce biases present in training data.
- Responsibility: Establishing who is responsible for decisions made by AI systems, especially in cases of negative outcomes.
Future Outlook on Responsible AI
The future of Agentic AI lies in responsible development and implementation. Key components of this future include:
- Inclusive AI Design: Engaging diverse stakeholders in the development process to ensure various perspectives are considered.
- Regulatory Frameworks: The establishment of regulations that govern AI use, emphasizing ethical practices and accountability.
- Continuous Monitoring: Employing mechanisms to monitor AI systems post-deployment to ensure ethical compliance and performance quality.