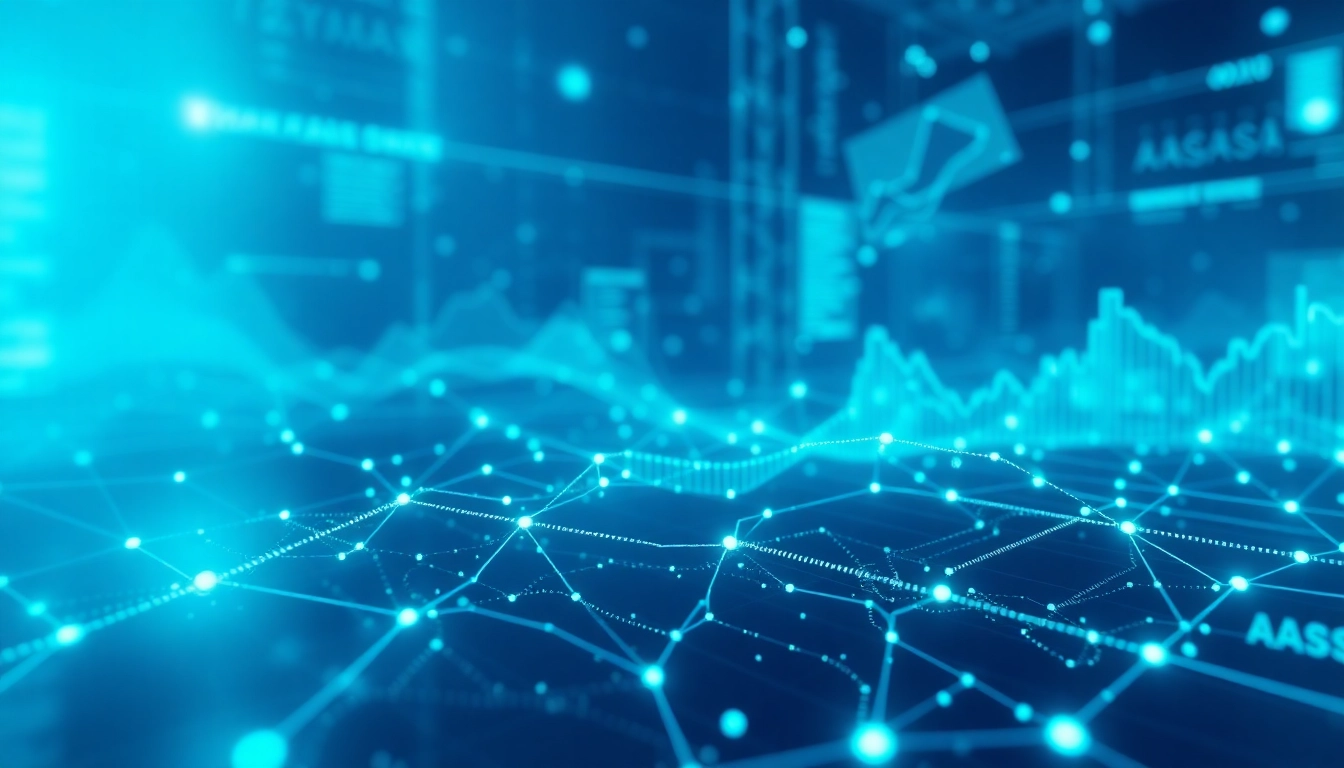
Understanding AI Blockchain Analytics
What is AI Blockchain Analytics?
Artificial Intelligence (AI) and blockchain technology are rapidly transforming the landscape of data management and analysis. AI blockchain analytics involves the use of AI techniques to extract insights from blockchain data. This hybrid approach enables organizations to efficiently analyze vast volumes of data available on the blockchain. By harnessing the capabilities of AI, such as machine learning and natural language processing, businesses can uncover patterns, detect anomalies, and generate forecasts based on historical blockchain transactions.
In essence, AI blockchain analytics combines the decentralized and secure nature of blockchain with the computational power of AI to provide more insightful analytics. This integration not only enhances the decision-making process but also promotes data-driven strategies across various industries. As organizations increasingly recognize the value of AI blockchain analytics, it becomes paramount to understand its significance, applications, and implications for future business operations. For insights on leveraging this innovative technology, explore AI blockchain analytics.
The Importance of Analytics in Blockchain Technology
Analytics play a vital role in enhancing the utility of blockchain technology. With blockchain’s inherent characteristics of transparency, immutability, and decentralized control, analytics helps to extract meaningful information that can drive business value. For instance, blockchain records contain a plethora of data regarding transactions, user interactions, and network activity. Using analytics, this data can be transformed into actionable insights that guide strategic initiatives.
Moreover, the importance of predictive analytics becomes apparent in the realm of risk management. Organizations can leverage AI algorithms to forecast potential threats, assess the viability of blockchain applications, and monitor compliance with regulations. By identifying patterns associated with fraudulent activities or accounting inconsistencies, businesses can safeguard their assets and enhance trust among stakeholders.
Key Components of AI Blockchain Systems
AI blockchain systems comprise several key components that work synergistically to facilitate efficient data analysis:
- Data Sources: Raw data from various blockchain networks, such as transaction ledgers, smart contract executions, and user interactions are primary input for AI models.
- Data Processing: AI algorithms process and clean the data, preparing it for analysis. This step often involves removing duplicates, normalizing data formats, and enriching datasets.
- Machine Learning Models: These models analyze the cleaned data to identify patterns, correlations, and anomalies. They learn from historical data to make predictions about future trends.
- Visualization Tools: Visual representations of data help decision-makers interpret complex datasets quickly, enabling faster insights and improved strategy formulation.
- Feedback Mechanisms: AI systems continually refine their predictions and models based on new data, leading to an evolving system that adapts to changing market dynamics.
Benefits of AI Blockchain Analytics
Enhanced Data Transparency and Security
One of the leading advantages of AI blockchain analytics is its contribution to enhanced data transparency and security. Blockchain systems are designed to be immutable and secure, which means that once data is logged into a blockchain, it cannot be altered without consensus from the network. By employing AI analytics, organizations gain an additional layer of security; AI algorithms can detect anomalies or unauthorized changes in data, triggering alerts and proactive responses.
Moreover, transparency is further enhanced as stakeholders can access and verify critical information. This level of transparency builds trust among users and partners, crucial for industries such as finance, supply chain, and healthcare, where data integrity is paramount.
Boosting Operational Efficiency with AI Insights
AI blockchain analytics can substantially boost operational efficiency by automating routine analytics processes. Traditionally, data analysis requires significant manual effort, resulting in time-consuming reports and potential human error. By integrating AI, organizations can automate these processes, allowing teams to focus on higher-value activities that require human intuition and creativity.
For example, automated reporting tools can provide real-time dashboard updates on blockchain transactions, supply chain movements, or financial status, enabling leaders to make quicker, well-informed decisions based on current data rather than relying on delayed reports.
Driving Better Decision-Making with In-depth Analytics
The depth of insights generated from AI blockchain analytics enables organizations to make more informed decisions. Predictive analytics can analyze past behaviors to forecast future trends and consumer preferences. This ability to anticipate changes helps businesses remain agile and responsive to market demands.
Furthermore, with detailed analytics, businesses can enhance their product offerings, tailor customer experiences, and optimize marketing strategies. In sectors ranging from finance to e-commerce, organizations that leverage thorough data insights enjoy a competitive edge.
Challenges in Implementing AI Blockchain Analytics
Technical Barriers to Adoption
While the benefits of AI blockchain analytics are compelling, organizations often face significant technical challenges during implementation. The integration of advanced AI capabilities with existing blockchain infrastructures can be complex and requires specialized skills. For many organizations, this represents a steep learning curve that may hinder timely adoption and effective utilization of analytics tools.
Moreover, the rapidly evolving nature of both AI and blockchain technology means organizations must continuously adapt and upgrade. Failure to keep pace with technological advancements can lead to obsolescence and inhibit performance gains.
Cost Implications and Resource Allocation
Implementing AI in blockchain environments can entail considerable investments. From the costs associated with new technologies, tools, and infrastructure to the need for skilled personnel, organizations must allocate resources judiciously. Smaller businesses, in particular, may struggle to justify the expenses linked to advanced analytics systems.
However, companies can mitigate these costs by engaging in partnerships with technology providers or utilizing cloud-based solutions, which often offer more affordable and scalable options compared to on-premises systems.
Data Privacy and Compliance Issues
Another notable challenge is navigating the complex landscape of data privacy and compliance. Blockchain, by design, ensures data immutability but raises questions about the compliance of retaining personal data with varying privacy laws such as GDPR or CCPA. Organizations must be wary of how they use and store data collected from blockchain transactions.
Implementing AI analytics without proper oversight can unintentionally violate privacy regulations, leading to legal consequences. To address these challenges, businesses must implement robust governance mechanisms, ensuring compliance from the outset of their analytics initiatives.
Best Practices for AI Blockchain Analytics Implementation
Identifying Relevant Use Cases
Before implementing AI blockchain analytics, organizations should identify pertinent use cases that align with their strategic goals. Assessing the specific challenges and needs within the organization can reveal opportunities for efficiency and growth. Use cases might encompass fraud detection, predictive maintenance, supply chain optimization, or personalized customer experiences.
By focusing on use cases that provide clear value, organizations can create targeted analytics initiatives that demonstrate measurable results and build momentum for broader adoption.
Choosing the Right Tools and Technologies
Selecting the appropriate tools and technologies is crucial for successful AI blockchain analytics. Organizations should evaluate their existing technological environments and identify compatible solutions that can integrate seamlessly with their blockchain networks. Familiarity with AI platforms, such as TensorFlow or PyTorch, along with blockchain platforms like Ethereum or Hyperledger, will aid in creating a robust analytics infrastructure.
Additionally, organizations can consider leveraging cloud-based analytics services that offer scalability, ease of use, and reduced upfront costs, allowing for more efficient resource use without straining IT departments.
Integrating Analytics into Business Processes
For AI blockchain analytics to yield real benefits, it must be integrated into business processes effectively. Start by aligning analytics initiatives with business strategies, ensuring that insights generated from data analyses are actionable and relevant to decision-making.
Moreover, creating a culture of data-driven decision-making within the organization is vital. Training staff on how to interpret and act upon insights will enhance the effectiveness of analytics tools while fostering a greater appreciation for data-driven strategies.
Future Trends in AI Blockchain Analytics
Increasing Automation and Machine Learning Integration
As technology continues to evolve, automation and machine learning will become increasingly integral to AI blockchain analytics. The convergence of AI with automation allows for the processing of data at unprecedented speeds, enabling real-time analytics that can significantly impact decision-making.
Organizations that capitalize on these advancements can streamline operations, reduce human error, and ultimately drive more innovative solutions that respond to emerging market trends.
The Role of Decentralized Data Models
The future of AI blockchain analytics will likely see a shift towards decentralized data models. Traditional centralized data repositories can introduce vulnerabilities, while decentralized approaches enhance security and availability. This shift will empower users to maintain greater control over their data while allowing AI algorithms to perform analyses in a distributed manner, providing more comprehensive insights.
As decentralized finance (DeFi) and decentralized applications (DApps) gain traction, the demand for decentralized analytics solutions will grow, pushing organizations to adapt and innovate in their analytics practices.
Anticipating Regulatory Changes in AI and Blockchain
As AI and blockchain technologies continue to proliferate, regulatory frameworks will evolve in response. Organizations must remain vigilant and adaptable to these regulatory changes, ensuring their analytics practices comply with new laws and guidelines. Proactive engagement with regulatory bodies and industry standards will be critical in shaping governance around AI blockchain analytics.
Moreover, understanding potential future regulations will provide organizations with opportunities to advocate for fair practices while contributing to the development of responsible innovation in the analytics space.